Statistical Learning Theory
Through research studies and projects on Statistical Learning Theory, sAIfer Lab optimizes model performances, balances ethics, and integrates physics-informed AI for robust solutions.
Statistical Learning Theory (SLT) is a critical framework in Machine Learning that guides the development and evaluation of predictive models.
It addresses key concepts such as hyperparameter tuning, which optimizes non-learnable model parameters through methods like grid search and Bayesian optimization, enhancing model performance.
SLT also focuses on generalization, ensuring models perform well on unseen data, with theoretical bounds like the VC dimension and Rademacher complexity providing insights into model capacity.
In addition to technical metrics like accuracy and precision, ethical metrics—such as fairness and transparency—are essential for responsible AI deployment.
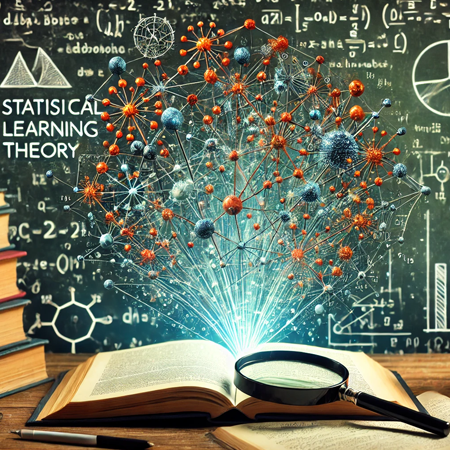
Emerging areas like physics-informed AI integrate domain-specific knowledge, leveraging physical laws to improve model robustness and interpretability. Future research in SLT aims to refine hyperparameter optimization, enhance generalization bounds, and establish standardized ethical evaluation frameworks, while expanding physics-informed AI to various scientific fields for more robust and interpretable models.
This comprehensive approach ensures that models are both technically sound and socially responsible, aligning with broader goals in AI development.
Active research projects
LAB DIRECTORS
Davide Anguita - Full Professor
Luca Oneto - Full Professor
RESEARCH DIRECTORS
Antonio Cinà - Assistant Professor
Luca Demetrio - Assistant Professor
FACULTY MEMBERS
Sandro Ridella - Emeritus Professor
PhD STUDENTS
Irene Buselli
Fabrizio Mori
Guido Parodi
Enzo Ubaldo Petrocco
Stefano Zampini
RESEARCH ASSOCIATES
Simone Minisi
Jinhua Xu