Intelligent Video Surveillance
sAIfer Lab develops Intelligent Video Surveillance solutions to support Law Enforcement Agencies in preserving people safety in public places.
sAIfer Lab works on the development of Machine Learning techniques for Intelligent video surveillance applications of computer vision, to support Law Enforcement Agencies (LEAs) in preserving people safety in public places.
We are investigating two specific applications:
Crowd counting and density estimation: during mass gathering events, LEA operators have to monitor in real time from control rooms a large number of videos coming from video surveillance camera networks covering public places (squares, streets, etc.), including airborne cameras (e.g., by unmanned aerial vehicles). Crowd counting/density estimation systems can support them by providing real-time estimates of the number of people in each video and of the corresponding density maps, which enables further functionality, e.g., automatically detecting events of interest such as overcrowding or other anomalous crowd behaviours.
Person re-identification: forensic investigations of incidents and crimes often include the analysis of a large amount of video surveillance footage to search for suspect individuals. Manual inspection is tedious and time consuming. Person re-identification systems support forensic investigators by automatically matching an image (query) of a suspect individual, selected by the operator in a video frame, against all the pedestrians detected in a given set of videos, based on clothing appearance. The operator is then returned a list of potential matches sorted for decreasing similarity to the query individual, from which the corresponding videos can be accessed for further inspection.
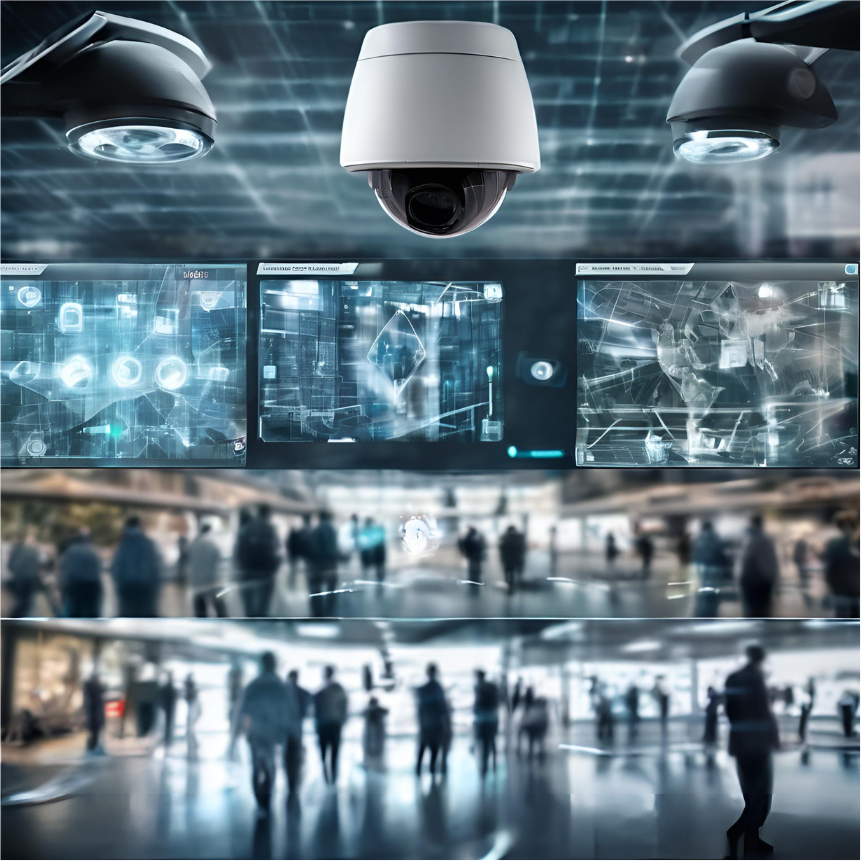
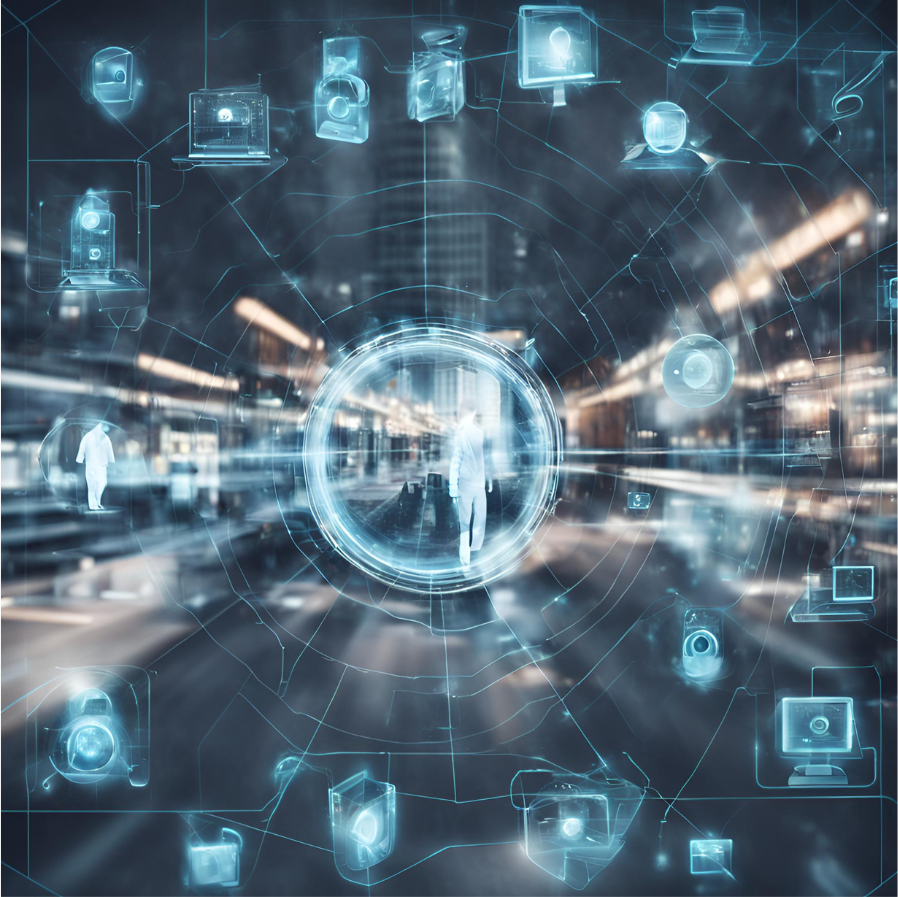
sAIfer Lab's contributions to this reasearch topic include:
for crowd counting/density estimation and person re-identification, we are investigating the use of synthetic images for training deep learning models, to avoid the need of collecting and manually annotating large amounts of video data, as well as privacy issues arising from the collection of images or videos showing individuals;
for person re-identification, we are investigating iterative improvement of the search results through a human-in-the-loop approach, by exploiting the operator’s feedback on the retrieved results (e.g., selecting true matches, and false matches either similar or dissimilar to the query individual).
RESEARCH DIRECTOR
Giorgio Fumera - Associate Professor
FACULTY MEMBERS
Lorenzo Putzu - Assistant Professor
POSTDOCS
Rita Delussu
PhD STUDENTS
Emanuele Ledda
LAB DIRECTOR
Fabio Roli - Full Professor